Benefits And Disadvantages Of Artificial Intelligence
페이지 정보

본문
By automating repetitive tasks, analyzing data rapidly and accurately, and optimizing general effectivity, AI brings substantial advantages to venture administration. Utilizing predictive analytics allows project managers to handle dangers proactive, while actual-time monitoring lets them spot points straight away. Task prioritization and scheduling are improved, streamlining workflows and boosting productivity. However, there are challenges, like potential preliminary implementation prices and concerns about job displacement. Successful and accountable integration of AI into challenge administration practices requires a steadiness between leveraging its efficiency gains and addressing these challenges. In conclusion, the advantages of artificial intelligence span several domains, together with automation, efficiency good points, and revolutionary options. From improved diagnostics in healthcare to optimized workflows in mission administration, AI brings important advantages. Nonetheless, it additionally comes with challenges together with, safety risks, moral concerns, and job displacement. Attaining a balanced integration of AI includes addressing these issues responsibly to unlock the complete potential of this transformative expertise. Join over 1000's of organizations that use Creately to brainstorm, plan, analyze, and execute their initiatives efficiently. Amanda Athuraliya Communications Specialist Amanda Athuraliya is the communication specialist/content material writer at Creately, on-line diagramming and collaboration instrument. She is an avid reader, a budding author and a passionate researcher who loves to write about all kinds of subjects.
This article will introduce you to various kinds of neural networks in deep learning and train you when to use which type of neural network for fixing a deep learning problem. It will even present you a comparability between these several types of neural networks in a simple-to-read tabular format! No sense of self: скачать глаз бога AI has no self-consciousness or self-driven creativity; the whole lot is programmed and may result in bias or inappropriate/dangerous outputs. AI is limited both by the data it’s trained with and the setting during which it’s working. AI bias: If coaching data will not be robust, correct, and diversified, the model can suffer from inaccurate or partial outputs. "They’ve simply been coded to place issues collectively which have happened collectively previously, and put them together in new methods." A pc is not going to on its own learn that falling over is bad. It needs to obtain suggestions from a human programmer telling it that it’s unhealthy. And likewise, machine learning algorithms will be lazy.
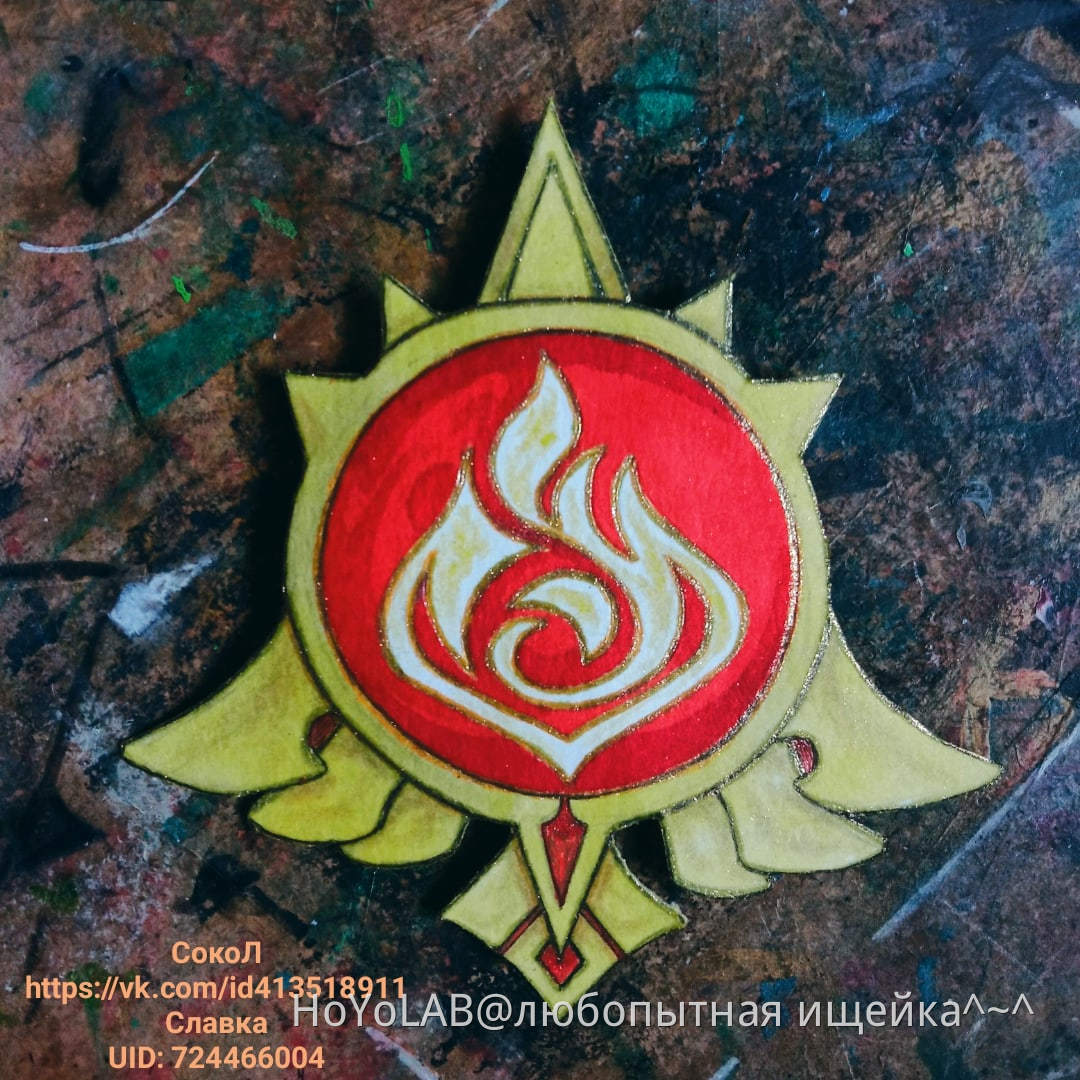
Recurrent Neural Community: The Recurrent Neural Community saves the output of a layer and feeds this output back to the input to better predict the result of the layer. The primary layer in the RNN is quite much like the feed-forward neural community and the recurrent neural network starts once the output of the first layer is computed. In the process of life individuals don't begin to suppose each second "from scratch". That's, the erasing of all previously accumulated data doesn't occur and any psychological activity relies on current information and expertise. All our knowledge and ideas are permanent. Traditional ANN should not have this property, and this is their principal disadvantage. It can be stated that the RNN builds dynamic models, that's, models that change over time in such a way that it is feasible to attain sufficient accuracy, relying on the context of the examples which were provided.
Classification - further layers serve as a classifier on top of the extracted features. These layers will decide the likelihood of how doubtless the image is being what the algorithm predicts it is. So, what are neural networks able to within the business setting apart from classifying information and recognizing patterns? Leaders from the AI analysis world appeared before the Senate Judiciary Committee to discuss and answer questions on artificial intelligence. Their broadly unanimous opinions typically fell into two classes: we have to act soon, however with a gentle touch — risking AI abuse if we don’t transfer ahead, or a hamstrung industry if we rush it. "Okay, that is all pretty fascinating, however where do Neural Networks find work in a practical state of affairs? Should you haven’t yet figured it out, then here it's, a neural network can do pretty much the whole lot so long as you’re in a position to get enough knowledge and an efficient machine to get the precise parameters. Something that even remotely requires machine learning turns to neural networks for help. Deep studying is one other domain that makes intensive use of neural networks. Activation Layer: By adding an activation perform to the output of the previous layer, activation layers add nonlinearity to the network. Pooling layer: This layer is periodically inserted in the covnets and its important operate is to scale back the size of quantity which makes the computation quick reduces memory and likewise prevents overfitting. Two common varieties of pooling layers are max pooling and common pooling. Flattening: The ensuing characteristic maps are flattened into a one-dimensional vector after the convolution and pooling layers so they are often passed into a totally linked layer for categorization or regression. Totally Connected Layers: It takes the enter from the earlier layer and computes the ultimate classification or regression job. Output Layer: The output from the totally linked layers is then fed into a logistic perform for classification duties like sigmoid or softmax which converts the output of every class into the chance rating of each class. Let’s consider a picture and apply the convolution layer, activation layer, and pooling layer operation to extract the inside characteristic. Load the picture and plot it. Apply convolution layer operation and plot the output image. Apply activation layer operation and plot the output picture. Apply pooling layer operation and plot the output image.
- 이전글The Honest to Goodness Truth on 스웨디시마사지 24.03.22
- 다음글다방 알바: Finding It Cheap 24.03.22
댓글목록
등록된 댓글이 없습니다.